산업 인력 감소가 전세계 추세로 관측되고 있는 가운데 물류 자동화를 도입하는 현장에 ‘물류 역동성(다이나믹스)’에 대한 대응 역량이 필수적으로 수반돼야 한다는 의견이 제시됐다.
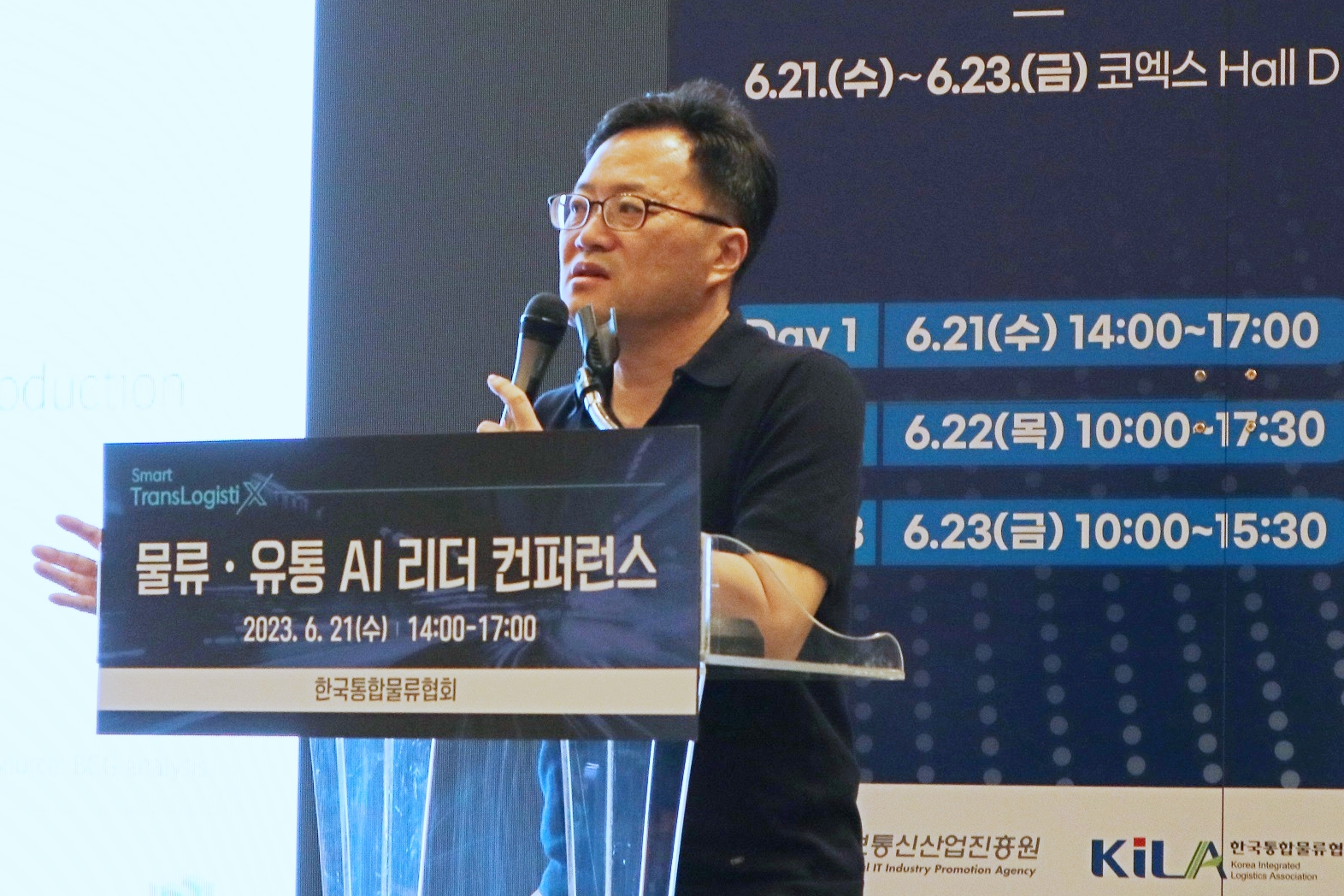
▲장윤석 한국항공대 교수
“배치-시리얼 공정 사이 다이나믹스 해결 必”
산업인력 5.4% 감소 추세, 도심지 60% 인구
아마존 키바 시스템, 오토스토어 등 물류AI↑
물류 혁신의 필요성은 지속해서 증가하고 있다. 산업 인력 감소가 전세계 추세로 관측되고 있는 가운데 물류 자동화를 도입하는 현장에 ‘물류 역동성(다이나믹스)’에 대한 대응 역량이 필수적으로 수반돼야 한다는 의견이 제시됐다.
21일 서울 삼성동 코엑스에서 정보통신산업진흥원과 한국통합물류협회 주관 ‘물류·유통 AI 리더 컨퍼런스’가 개최했다.
이번 행사는 AI 및 빅데이터를 활용한 물류산업의 미래와 스마트 모빌리티 분야를 물류산업에 융합해 나아갈 방향으로 탐구하는 자리로, △AI를 활용한 물류 혁신의 방향 △2023 물류 AI 기술 융합 트렌드 △챗GPT와 스마트 물류 모빌리티 주제가 각각 3일 간 진행된다.
첫날 장윤석 한국항공대 교수는 물류 AI 기술 도입의 선결 조건과 과제를 발표하며 물류에서의 ‘다이나믹스(Dynamics, 역동성) 프로세스’의 중요성을 강조했다. 물류의 역동성 측면은 시리얼-배치 공정 간에 연결 등에서 고려해야 핵심 요소이다. 시리얼(Serial) 공정은 1번에 1개의 작업을 수행하고, 배치(Batch) 공정은 1번에 여러 개의 작업을 동시에 수행하는 일괄 처리를 일컫는다.
장 교수는 “배치 공정에서 물건이 나올 때는 한꺼번에 나온다”며 이후 시리얼 공정이 이어질 경우 병목 현상 등의 이슈가 발생할 수 있다는 점을 설명했다. 이에 “공장과 물류 현장에선 다이나믹스 공정 해석에 한계가 존재한다”며 “공정상 이러한 부분을 고려할 필요성이 있는데 현장은 시스템 구축에 대한 역량이 부족하다”고 지적했다.
이를 위해선 AI 알고리즘과 자동화 시스템 구축 시 이러한 다이나믹스 해석에 지식과 역량을 갖춘 개발자 및 현장 관리자들이 협업하는 것이 무엇보다도 중요하다.
전세계적으로 산업인력이 감소하는 추세선이 지속되고 있으며, 장 교수는 보스턴 컨설팅 그룹(BCG) 자료를 인용해 “2030년이 되면 산업 인력풀이 5.4% 줄어들며 전세계 인구의 60%는 도시 지역에 살게 될 것”이라고 예측했다.
장 교수는 물류 자동화에 사용되는 자율 주행 모빌리티 기술인 AGV(Automated Guided Vehicle)와 AMR(Autonomous Mobile Robots)는 각각 장단이 명확해 AMR의 경우 “평탄도가 좋지 않으면 장애물로 인식할 수 있으며, 사람과 함께 작업하는 공간 및 테이블 다리가 많은 곳 등에선 너무 많이 멈춰 작업 효율이 20% 남짓에 불과하다”고 강조했다. AMR은 정밀도도 떨어지는 만큼 제조 공정에서의 물류 자동화로 활용하기는 현재로는 어려운 형편이다.
또한 그는 “공간 활용성과 밀집도가 뛰어난 오토스토어의 솔루션은 도심지 공간이 부족한 물류 창고에서의 특정 물류 애플리케이션에선 활용성이 기대된다”면서도 “물동량이 많고 너무 무거운 제품들이 많은 곳에는 적절하지 않다”고 언급했다.
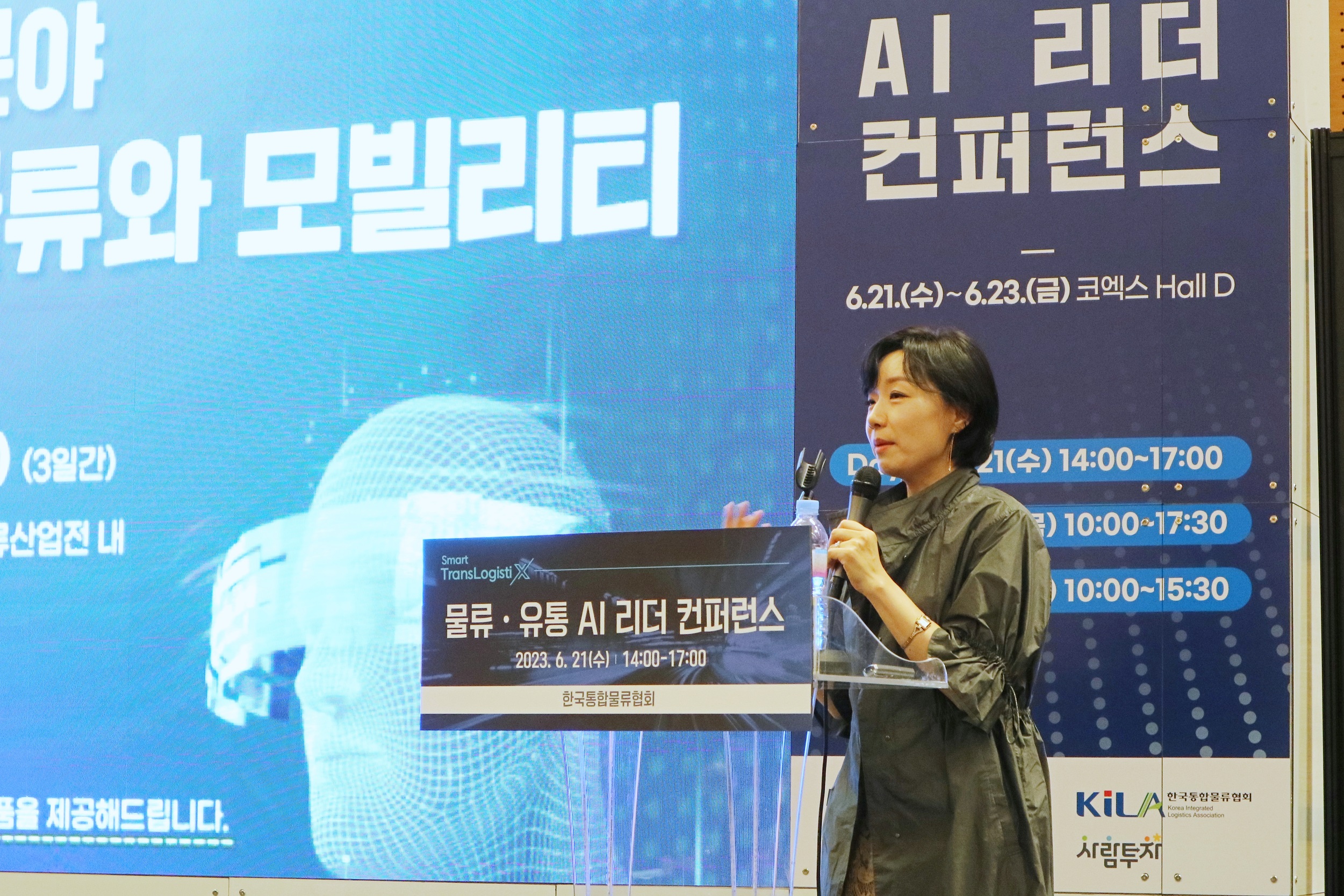
▲손병희 국민대 교수
AI 혁신과 물류·모빌리티 융합 방향을 설명한 손병희 국민대 교수는 “물류와 모빌리티 분야에서 AI 활용의 중요성은 △실시간 모니터링과 의사 결정 지원 △작업자와 사용자 등 고객 경험 향상 △유연성 △자동화 △미래 예측 등에 있다”고 말했다.
빅데이터를 활용한 딥러닝, 머신러닝으로 미래 예측이 현장에 적용될 경우, △재고관리 △창고 운영 등 물류 네트워크 최적화가 이뤄질 수 있으며 이미 이러한 기술들이 아마존 등 대형 물류기업을 중심으로 도입되고 있는 추세이다. 대표적으로 오토스토어의 물류 자동화 솔루션이나 아마존이 구축한 키바 시스템 등이 그 예이다.
향후 AI가 맞이하게 될 도전과제로 △학습 데이터의 품질과 가용성 △AI인력 기술 역량 △데이터 보안과 개인정보 보호 △윤리적 문제 발생 등을 고려할 필요가 있다고 지적했다.